Robot navigation has become more and more advanced in recent years, but in very crowded environments, such as public areas or roads in urban environments, most robots still cannot navigate accurately. In order to be widely used in smart cities in the future, robots need to be able to navigate reliably and safely in these environments without colliding with humans or nearby objects.
Image source: Martinez-Baselga, Riazuelo & Montano
The collision trajectory of the robot trained by standard exploration strategy (left) and the successful trajectory of the robot trained by intrinsic reward in the same scene.
Researchers from university of zaragoza and Aragon Institute of Engineering recently proposed a new method based on machine learning, which can improve robot navigation in indoor and outdoor crowded environments. This method is used inarXivAccording to a paper published in advance on the server, it is necessary to use intrinsic rewards, which are essentially "rewards" that AI agents get when they perform behaviors that are not strictly related to the tasks they try to complete.
Autonomous robot navigation is an unresolved problem, especially in unstructured and dynamic environments, robots must avoid colliding with dynamic obstacles and achieve their goals.
Facts have proved that the deep reinforcement learning algorithm has high performance in success rate and time to reach the goal, but there are still many places to be improved.
The method introduced by Martinez Baselga and his colleagues uses intrinsic rewards, aiming at increasing the motivation of agents to explore new "states" (i.e. interactions with their environment) or reducing the level of uncertainty in a given scenario, so that agents can better predict the consequences of their actions.
In their research background, researchers specially use these rewards to encourage robots to visit unknown areas in their environment and explore their environment in different ways so that they can learn to navigate more effectively over time.
Compared with the same algorithm with ICM (intrinsic reward), the training index of the most advanced algorithm.
Most deep reinforcement learning for crowd navigation focuses on improving the processing of network and robot perception.
My method studies how to explore the environment during training to improve the learning process. In training, the robot does not try random movements or optimal movements, but tries to do what it thinks it can learn more from them.
The researchers evaluated the potential of using intrinsic rewards to solve robot navigation in crowded spaces in two different ways. The first one integrates the so-called Intrinsic Curiosity Module (ICM), while the second one is based on a series of algorithms called Efficient Exploratory Random Encoder (RE3).
The researchers evaluated these models in a series of simulations, which were run on the CrowdNav simulator. They found that their two methods of integrating intrinsic rewards are superior to the most advanced navigation methods of crowded space robots developed before.
In the future, this research can encourage other robotics experts to use intrinsic rewards when training robots to improve their ability to cope with unforeseen situations and move safely in a highly dynamic environment.
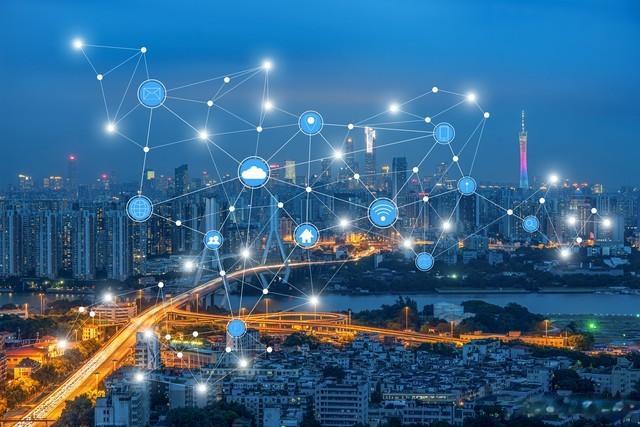
In addition, the two models tested based on intrinsic rewards will soon be integrated and tested in real robots to further verify their potential.
The results show that by applying these intelligent exploration strategies, the robot can learn faster and the final learning strategy is better. And they can be applied to existing algorithms to improve them.
In the next research, it is planned to improve the deep reinforcement learning in robot navigation to make it safer and more reliable, which is very important for using it in the real world.
关于作者